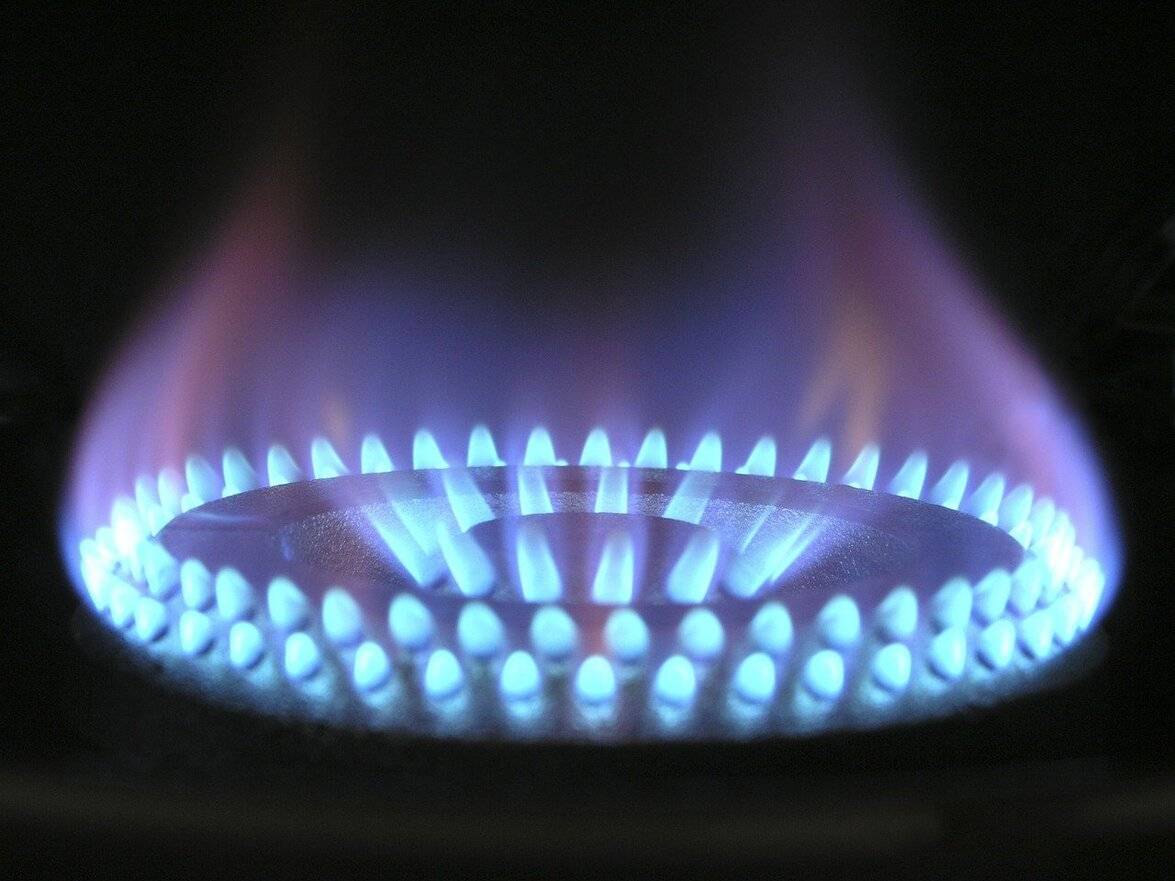
Efficiency Savings
Machine Learning in the Oil and Gas Industry
Read a summary or generate practice questions using the INOMICS AI tool
Machine learning is the ability of an algorithm to learn from data and improve its accuracy over time. It is a concept that is on everyone’s minds in today’s technological, innovative world.
Machine learning can be used to find patterns in data, which can then be used to make decisions and predictions. The algorithm used to do this gets more accurate as it processes more data. Machine learning is especially useful with data sets that are too large to analyze manually. More accurate results mean more efficient processes, which have benefits for safety, people, the environment, production, and profits.
Diverse fields, such as the finance and transportation sectors, are coming to rely more on machine learning as a way to improve and refine operations. The oil and gas industry is starting to adopt it too, but as of writing it is not yet commonplace. The following explores how the concept could be more readily applied, and the advantages, both financial and environmental, it could bring.
Why should the oil and gas industry use machine learning?
As the global population increases, so will energy demand. Although a global shift toward renewable energy sources has started, and should be prioritised, oil and gas will provide the world with some energy for the foreseeable future. In 2016, 55% of global energy demand was supplied by the oil and gas industry, and by 2040, it is anticipated that that number will increase to 57%.
While a complete switch to renewable energy sources would be best for the environment, that reality, unfortunately, is still some years off. In the meantime, as the transition is underway, machine learning should be deployed in oil and gas to help improve safety and efficiency in the industry’s coming years.
Not only could it ensure that energy is produced as efficiently as possible, it would reduce the industry’s environmental impact and mean that higher volumes of energy could be produced at a lower cost than ever before. But how?
How can machine learning be used in the oil and gas industry?
Because of the number of processes associated with oil and gas production, machine learning has many possible uses in this industry. Some examples are included below.
Predictive maintenance
In many companies, maintenance is addressed on a reactive basis rather than a proactive basis. Equipment is repaired when it fails instead of maintaining it before it fails. A reactive approach can lead to damaged equipment and unexpected downtimes.
Machine learning can help companies make the switch to predictive maintenance by modelling sensor data to find problematic equipment. If there are anomalies in the dataset, such as equipment operating outside its parameters, then the equipment can be maintained before it is damaged. Damaged equipment leads to safety issues and reduced production.
Predictive maintenance can also reduce environmental impact. Well-maintained equipment fails less, so fewer spills happen. Spills in the industry can be almost impossible to completely clean up and can have far-reaching effects on people, water, animals, and soil.
Data trending
Machine learning can be used to trend data to determine optimal operating conditions. As an example, take steam injection, where steam is injected into an oil reservoir to heat it, making the oil less viscous.
Engineers are responsible for determining the flow rate and temperature of that steam. They must identify which combination of steam flow rate and temperature bring the most oil to the surface. The optimal combination can change daily. Instead of having to continuously test combinations, machine learning could be used to model the reservoir and calculate the optimal combination, saving time and money.
Well logging
Well logs are produced when a measurement probe is lowered into the wellbores of oil wells. These logs are used to collect information about the surrounding environment, including well depth and the amount of oil in the reservoir (known as oil in place).
In an area where no wells have been drilled, an exploratory well is used to see if there is oil or gas in the area. If an exploratory well is discovered to have oil or gas, more wells, called development wells, are drilled because the area has been proven to have oil or gas.
Economics are a major driver in the oil and gas industry. If an oil well is not profitable, the operator will usually choose to abandon it. Development wells don’t always live up to expectations.
Machine learning can help to more accurately determine the production potential of a well. With the more accurate predictions produced by machine learning, significantly less money is wasted if a development well is abandoned.
Despite a worsening global climate crisis, oil and gas companies will continue to drill wells until stricter regulations are enacted and oil and gas companies are priced out of drilling. Because machine learning helps to select better wells, fewer wells destined to be abandoned would be drilled. There would be no need to worry about emissions produced from these wells or any spills if they were never drilled.
Warning systems and anomaly detection
Contaminants in oil wells are a major problem in the industry. They can plug the wells, leading to a slowdown in production. Machine learning can be used to model contaminant concentrations and identify the source of a problem.
Machine learning was used for just this purpose off the coast of Norway, for example. Chalk silt in the seawater was plugging the wells, and the models that were in place were not specific enough to make accurate predictions about future clogs. Machine learning was used to develop a better model so that chalk buildup could be identified as soon as it started. This kept production rates up and kept equipment undamaged, saving the well operator up to $15 million a year.
Risk identification and mitigation for field workers
The oil and gas industry is full of hazards, especially for frontline field workers. They can be exposed to toxic gases, for example, or be caught in machinery.
Luckily, machine learning can be employed to mitigate these risks. How risk presents itself in the field can be understood, which allows for potential sources of risk to be identified. These sources of risk can then be addressed to maximize safety potential. For example, machine learning could monitor video footage to see if there was somewhere where leaks kept forming. A better protocol could then be developed to address those leaks, which would safeguard field workers.
Energy efficiency
It takes energy to produce oil and gas, and lots of it. The steam used in injection wells, for example, is produced using natural gas as a feedstock. Electricity is produced by driving turbines with steam, which is again produced using natural gas.
Unfortunately, energy efficiency isn’t at 100% in the industry. There is a lot of wasted energy, such as by letting heat escape in a process or by flaring and venting greenhouse gases.
Machine learning can help to reduce inefficiencies and wastage by pinpointing deficiencies. For example, sources of energy waste, such as heat from an oil reservoir not being used in the oil treating process, can be identified and corrected so that energy can be used towards producing more oil or gas. Fewer greenhouse gases would be vented, which would improve air quality.
Equipment inspection
Equipment must be inspected regularly to ensure that it is in good shape. Although necessary for safety and integrity concerns, these inspections can take a lot of time that could be better used elsewhere.
Take the inspection of pipelines as an example. Someone must walk along the length of a pipeline to check it for anomalies, which can take many hours. A drone, on the other hand, could inspect the pipeline in less time. But drones aren’t always as good as humans in following the length of a pipeline. Using machine learning to identify the exact route of a pipeline would ensure that the drone could properly inspect the pipeline, allowing a human operator to have more time to do other tasks.
Identifying oil seeps
When crude oil leaks from breaks in the seafloor, an oil seep has occurred. This oil is difficult to remove from the water and has implications for marine life and the environment.
Machine learning can help to detect these seeps so that their impact can be minimized. In 2016, artificial intelligence robots were used by ExxonMobil to detect oil seeps. Because of this, exploration risk is lowered significantly, and harm to marine life is lessened.
Implications for the oil and gas industry
Whether on or offshore, machine learning has significant implications for all aspects of the oil and gas industry. This industry will be a major player for years to come because of the slow shift towards renewable energy. To help with reducing environmental impact and safety concerns, therefore, it is in everyone’s best interest—the public, oil and gas companies, and the environment—that the use of machine learning in the oil and gas industry becomes widespread.